Through UROPs, Students Explore Topics in Sustainability Relevant to Their Interests, MCSC, and Member Companies
September 21, 2021
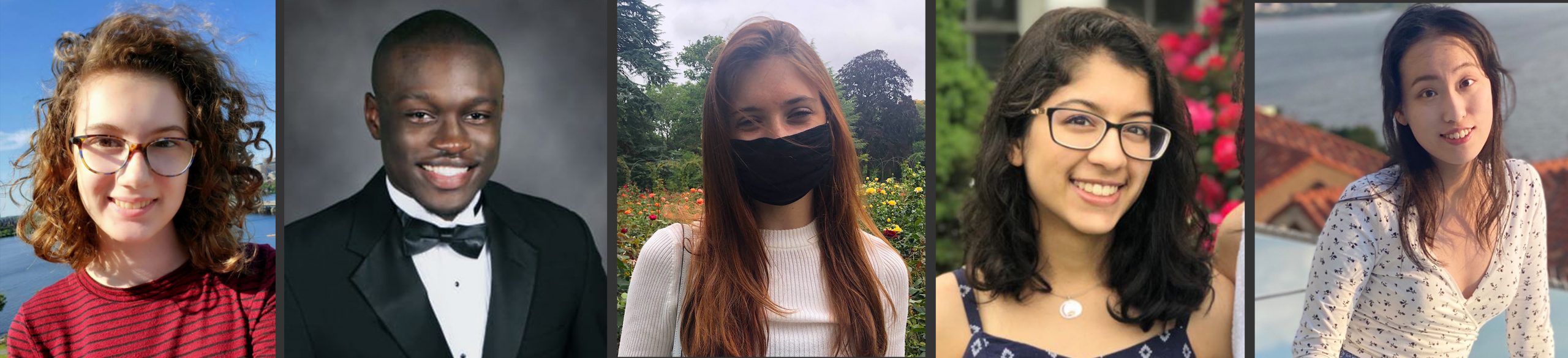
For many students, the summer months presented an opportunity to dive into research projects through the MIT Undergraduate Research Opportunities Program (UROP). This was also a chance for the MIT Climate & Sustainability Consortium (MCSC) to support a cohort of students embarking on UROP projects that engaged with a climate and sustainability topic related to the Consortium, and in some cases, one of its member companies. This inaugural cohort of MCSC UROPs explored topics including minimizing greenhouse gas release, developing green hydrogen fuel cell models, exploring the social costs of climate change, assessing the state of health of spent batteries, and investigating game development for traffic congestion mitigation. Congratulations on a successful summer of research; learn more about each of the projects below.
Katherine (Katie) Shaw (Wellesley College) spent the past several months exploring the social costs of climate change, and estimating the impact of extreme temperatures on human behavior and sentiment. The reduction of threatening effects of temperature on the health and well-being of citizens is a major driver of environmental regulation for most countries around the world. Extreme temperatures poses a serious threat to the functioning of the human brain, hindering cognitive performance of individuals and the productivity of cognitive workers. In their project, the team has been using a unique global dataset to investigate how air temperature affects the behavior of individuals in a variety of behavioral experiments. Working with Dr. Juan Palacios and Dr. Siqi Zheng, Faculty Supervisor and also a member of the MCSC Faculty Steering Committee (FSC), Katie has focused on data processing and analysis as well as literature review.
“My goal going into this project was to learn more about how extreme temperature is affecting human populations,” said Katie, who has been examining weather information coming from the Integrated Surface Database (ISD) – from the National Ocean and Atmospheric Administration (NOAA); the ISD holds various information in areas such as temperature, visibility, wind, and sky conditions. She has then been matching this data with behavioral experiments and measures of sentiment (such as Prisoner’s Dilemma, or Public Goods Games).
“Previous findings support that exposure to extreme temperatures and extreme temperature can affect long-term academic performance, and extreme temperatures can also impact decision-making, human behavior and sentiment,” explained Katie. “As a next step, we may explore weather filtering and checks, conduct temperature analyses, and look at the effect on the cognition of decision-makers.”
Louise Anderfaas (MIT, Materials Science and Engineering) has been working alongside Dow, MCSC member company, to strategize on minimizing greenhouse gas (GHG) release. She spent much of her time compiling information and considering the benefits and drawbacks of using hydrogen, and how to make it viable. As Louise describes, “In recent years, hydrogen has attracted renewed attention for being a method of decarbonization.” Today, many countries have hydrogen roadmaps, and there are hundreds of hydrogen projects under development with substantial public funding.
In exploring the main question of why we should be using hydrogen, Louise compiled and read relevant literature, visualized the relevant information, and conducted informal calculations wherever she could. Her results were mixed, and, in her own words, “there is not currently an answer to every decarbonization need.” She found that it is most promising in hard-to-abate sectors, in more self-contained industries, like the high temperature industry and shipping. Monetary concerns and large-scale coordination remain primary challenges moving forward.
As a next step, Louise poses looking at hydrogen from a user-based perspective using a decision tree mapping, considering various hydrogen use-case scenarios, as well as investigating potential uses for by-product oxygen.
Joshua Kuffour (MIT, Chemical Engineering) has been working with MathWorks, MCSC member company, to develop green hydrogen fuel cell models using Simulink (a software that allows users to design and simulate their system before moving to hardware) and MATLAB (a programming and numeric computing platform that analyzes data, develop algorithms and create models). Differing from Louise’s project, Joshua took a more simulation and data-driven approach by focusing on green hydrogen and modeling microgrid structures, incorporating elements of solar power and storage, and making a reversible hydrogen fuel cell. As Joshua explained, “microgrid simulations are important because they serve as a basis for real-life renewable grids to be created, explain some benefits and drawbacks of renewable grids, are an inexpensive way of testing different operation conditions, and allow for intuition to be gained about what a real grid may need.”
Joshua’s work on the project spanned from the research phase (literature review on hydrogen fuel cell technology, MATLAB, and Simulink) to the implementation of new models. As he progressed, he kept track of their advantages and drawbacks, which considered aspects like efficiency, power, duration, and storage capability, and has been working to interpret these models in the context of industry and governmental policy. Through their key findings, four models were created: constant irradiance, no resistance, gas modeling, and moist air modeling (most complex) – and there are many next steps to this research. Thinking ahead, the fidelity of the moist air model can be increased in many ways, energy storage options can be added to the model, and there are various operational scenarios to test and expand. In addition, there are lots of potential crossover with Louise’s project as they aid in discussing which color of hydrogen should be pursued by backing it up with operational scenarios.
Elliott Seaman (MIT, Architecture) has also been working with MathWorks, but to develop computational methods for modeling energy usage and physical properties of building climate control. Using Simulink and MATLAB, the main goal has been to set up a framework, compilation of helpful resources, and simulation tool would allow future student researchers and outside collaborators to continue the work.
“I am very passionate about building technology,” said Elliott. “HVAC systems are a fundamental part of the built environment, and as global demand (especially for cooling) continues to increase, we are going to need greater energy efficiency moving forward.”
Elliott’s project started with a background research phase compiling reference documentation for people joining the project, and then moved into prototyping phase: creating a simulation in MATLAB/Simulink to give performance data for a selected building system,while also providing a substrate on which to build further complexity.
“Using the current framework, future explorations could include multi-room housing, including daylight, implementing more nuanced control systems and passive heating and cooling methods, expanding thermal properties to include more building materials, and modeling the money recouped by installing solar panels to harvest radiative solar energy,” Elliott concluded. “Exploring questions surrounding social-focused aspects and design justice would also be incredibly valuable.”
Grace Chang (Wellesley College) has been investigating game development for traffic congestion mitigation, with the goal to reduce carbon dioxide (CO2) through reduced congestion. Working under MIT Professor Cathy Wu, Principal Investigator, Grace has been aiming to extract driving strategies employed in autonomous vehicles (AVs) and apply them in human driving contexts. Previous findings suggested that AVs greatly alleviate congestion, but since they might not be prevalently used for another many years, it is important to first apply their general approaches to mitigate congestion.
Grace’s main role throughout the project has been to create a game in Unity, a video game software, that simulates a real-time and realistic driving environment (e.g. one that includes acceleration) to teach users about various AV strategies in the future. The first version of the game included a circular track and used the intelligent driver model (IDM), a time-continuous car-following model for the simulation of freeway and real-time urban traffic environments. It is key that the game accounts for both acceleration and deceleration. The next version would incorporate Python, a powerful programming language, to allow for integration with a physical driving simulator.
Anusha Puri (MIT, Biological Engineering) has been working to develop an algorithm to quickly assess the state of health of spent batteries, and to determine if they should be reused or recycled. A low-cost technique to determine the status of a secondary battery is electrochemical impedance spectroscopy (EIS). The spectra and signals obtained from EIS can be represented using an equivalent circuit model (ECM). Currently, an algorithm enabling the generation of an EIS curve from a circuit model exists. Working with MIT Research Scientist Juner Zhu (principal investigator), Wei Li (postdoc), and Professor Maria Yang (a member of the MCSC FSC), Anusha’s role has been to develop a machine learning program to address the reverse problem, of identifying key parameters of the circuit model based on the EIS curve.
In her role, Anusha has been learning the EIS method of battery assessment and using the equivalent circuit model to generate artificial EIS curve data, developing a machine learning based program to identify parameters of the equivalent circuit model from EIS curves, finding the minimum number of data points needed in an EIS curve to identify the above parameters with acceptable accuracy, and testing the model by creating artificial EIS curves with noise and using the model to classify the status of health (healthy or damaged). Her goal has been to develop a machine learning based program that can accurately identify key parameters of the equivalent circuit model (ECM) based on electrochemical impedance spectroscopy (EIS) curves. Following this, the model should be able to accurately and efficiently classify battery status.